AI Tools For Developers: Essential AI-Powered Solutions For Modern Dev Teams
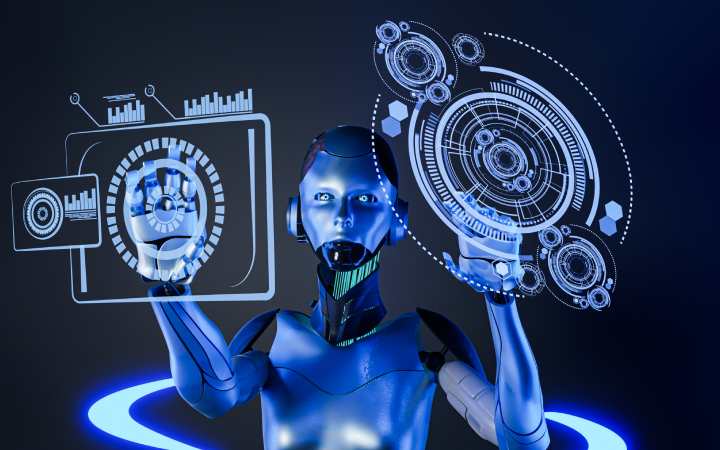
Automation and artificial intelligence are the trends that are reshaping today’s fast-growing world of software development, helping introduce intelligence into the development process, predicting the further development of the program, and offering recommendations. These tools reduce time consumption by optimizing repetitive work, highlighting possible risks that might appear to be productive work but are, in fact, fatal, and work on the overall enhancement of the product.
AI can be beneficial in improving development productivity, reducing the time spent on error debugging, and cutting down the time to develop products. Because of the overall demand for very fast software delivery of high quality, AI tools for developers have shifted from being simply nice-to-have, but rather have become fundamental parts of a modern software development process to sustain the competitiveness of the teams.
Why AI Tools Are Essential For Developers
AI-powered tools are transforming every stage of the software development lifecycle, from coding and debugging to testing and deployment.
AI-Powered Code Assistants
Coding companions in the form of GitHub Copilot, Tabnine, Kite, etc. include features such as code recommendations and completions, and intelligent tracing. Information Technologies tools assist developers in writing more efficient syntax and provide suggestions based on the standards. By using deep learning models based upon large code repositories, these assistants increase productivity because they alleviate the cognitive work of developers.
They also facilitate the generation of contextual codes, which are essential for the development of prototypes as well as code rewriting. Moreover, AI assistants are quite compatible with most of the common IDEs, enabling them to be a useful tool not only for scripters within the chosen programming language but also for specialists in other programming areas.
AI-Based Bug Detection and Debugging
There are sites like DeepCode, Codacy, and Sonar Qube which employ Artificial Intelligence to analyze code quality, identify issues such as vulnerabilities, and compliance with coding standards. These tools help maintain the code integrity and security, and also minimise the efforts which will be required for the manual review. It automates reviewing by analyzing code and comparing it with known inefficiencies, security vulnerabilities, and other possible glitches that could be fatal to the application.
Since it builds on the previous feedback received and the experience of software engineers on the subject, these tools allow the identification of actions that will enhance the maintainability of software. They also work with CI/CD, which means that quality assurance for green software is carried out continuously throughout the development process, improving the relations between development teams.
AI-Powered Testing Frameworks
Some testing tools are Test.ai, Functionize, and Applitools; these tools involve the use of artificial intelligence for testing. It enhances the coverage into tests in a way that it recognises the modifications done on the user interface, estimates areas that may fail the tests, and defines the most optimal ways and environments to run the tests in. These tools help in augmenting the visual testing process using machine learning that assists in identifying falsehoods quickly by checking that all UI elements are pixel-perfect across different devices and browsers.
Self-Healing Coded Testing is another testing framework; it allows the integration of artificial intelligence to determine the impact made to a User Interface in the test scripts to fix it on its own, and make the tests more resistant to failure in the end. There are testing tools that employ the use of artificial intelligence, for instance, Test.ai, Functionize, Applitools, among others. They enhance coverage since they have a way of detecting new changes in the User Interface, predicting test failures, and also taking into consideration the environments in which the tests should run.
AI For DevOps And CI/CD
Some of these are cloud-based smart DevOps tools that enable the probable tools for deployment, infrastructure monitoring, alarms, and performance, such as Harness, CloudBees, and Dynatrace. These tools guarantee and deliver integration while it’s in process with the least amount of human interaction. Real-time monitoring of a system is possible through AI-driven monitoring solutions for detecting signs of performance issues and forecasted system failure, and offering recommendations to avert such breakdowns.
Also, the AI in CI/CD systems tracks resource consumption to help in predicting and minimizing build failures and enhancing the use of resources in deployment. These tools make the core DevOps activities more scalable, secure, and reliable in the process of software development. The list of AI-powered DevOps tools includes Harness, CloudBees, and Dynatrac, which help provide the intelligence for smart deployment pipelines and enable monitoring of the infrastructure, detecting issues, and analysis of deployment performance. These tools ensure perfect compatibility and a constant building/ deployment process without the need for interaction.
AI In Natural Language Processing (NLP) For Code Documentation
OpenAI’s Codex and Amazon CodeWhisperer can assist developers in generating usual comments and precise and detailed documentation connected with the concepts that may be progressively difficult for an ordinary user to comprehend concerning the architecture of the program code. These combine NLP functionality to understand context based on the code and come up with accurate documentation to help with knowledge transfer and better code maintainability.
They help to separate the issue of developers spending a lot of time on writing explanations and make codebases more accessible for the teams that work on large-scale projects. Further, these AI techniques help developers in the onboarding process by giving them detailed descriptions with regard to functionality, dependencies, and logic of the code representing the new projects they are going to join.
Benefits Of AI Tools For Development Teams
AI technologies are gradually becoming pivotal to software development by reducing the amount of manual work and offering high-quality code, resulting in faster debugging. These intelligent solutions increase efficiency during teaming, improve the work processes, and free developers from solving recurrent tasks rather than spending their time on developing ways of solving more complex problems.
Improved Efficiency: AI can suss out reinvention of similar programs, hence freeing the developers to work on new concepts rather than rewriting code most of the time. It increases efficiency and enables development processes to be accomplished in a shorter time, hence its advantage of accelerating project delivery.
Better Code Quality: Recommendations involve updates regarding the code structure, its maintainability, and its security since the AI can detect the issues with the code. These tools use machine learning algorithms to identify loopy code fragments and alert the developers by identifying the shorter and recommended patterns.
Faster Debugging: Automated bug tracking can decrease the debugging process time and speed up the release and delivery of stable programs. There are AI-powered tools that consider error logs, trace through stack flows, and distinguish similar problems with different classifiers. These tools have the feature to sort the bugs based on the priority, such as the importance of the bug and how frequently it occurs, so that the main problematic bugs can be fixed first.
Scalability: AI solutions for DevOps will help them to predict and resolve issues affecting application scalability while assuring that the scalability of the system and application does not compromise the availability of applications.
Better Collaboration: AI technologies reduce the number of potential issues with code reviews, share information about defects and best practices with multiple teams, and make suggestions and provide feedback in real-time while integrating with collaboration platforms. Such tools help developers to become more efficient and to produce a cohesive code base as they provide suggestions, mark potential problems, and provide recommendations in regards to the existing code standards.
How LambdaTest Leverages AI For Smarter Testing
AI application integration in software testing ushers in a new way of testing that is much faster and more accurate as compared to the traditional methods of testing. One of the leading contenders in this revolution is ‘LambdaTest’, it is a cloud-based cross-browser testing AI tool that was designed to facilitate the entire process of testing websites and ensuring better responsiveness on as many devices as possible.
It does so through a full-featured test suite, which allows developers to check whether their site is optimized and accessible to all visitors across the various devices and browsers they are likely to use. LambdaTest is an AI-native test orchestration as well as execution tool that can perform various tests on more than 3000+ browser-OS combinations, including 10,000+ real environments.
AI-Native Test Execution
LambdaTest uses AI for test orchestration in order to execute the tests on different browsers, devices as well and environments. Hence, by achieving test distribution & parallel execution, LambdaTest decreases the test execution time & status test coverage. This is because AI places emphasis on high-risk sections to avoid congestion in testing cycles. RIGI has cited that one of the major difficulties in automated testing is script maintenance.
Self-Healing Test Automation
The self-healing process is an AI-native mechanism that can update the test scripts in collaboration with the UI elements that change frequently, thus decreasing flakiness and manual interferences. This feature is to keep the test stable and ensure the test automation is consistent over time.
AI-Native Visual Testing
With the help of AI, LambdaTest is smart enough to perform the visual regression testing to identify the differences in UI during the different runs. AI scans the pixel level of the page layout and compares colors and designs across different devices and web browsers to find out layout changes, color differences, and ensure that there is no mismatch in design layouts.
Conclusion:
The application of AI in software development is rapidly advancing to reduce the role of software developers by automating, analyzing, and solving problems. These tools help in automating repetitive work and also give details about the standard of the code, security troubles, and even ways for the application to execute optimally. Advanced algorithms enhance the working process organization by analyzing possible problems that should be expected in a working process, pointing out the discrepancies in the working process, and providing recommendations on how to avoid them in the future.
These labs include code assistants that suggest intelligent code to developers, test automation frameworks that enable the development of highly reliable applications, among others, which help development teams to write and test applications in record time and with precision. This paper aims to show that by incorporating AI into the team’s toolkits, current software development teams can shorten development cycles and improve the flow of the work of developers as well as enhance the attitude toward innovation.